Revolutionizing Data Annotation: The Power of Video Labeling Tools in Machine Learning
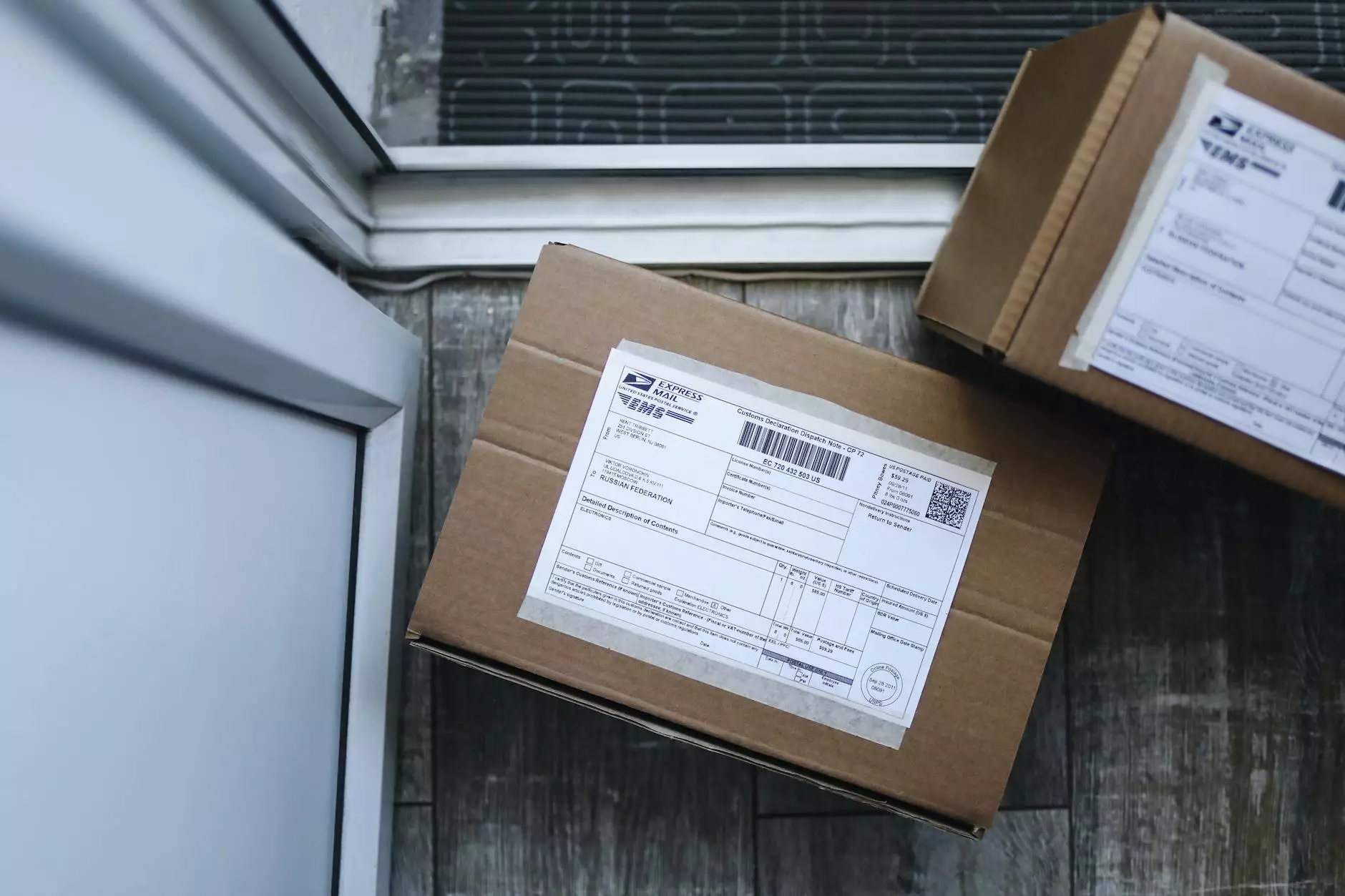
In today's fast-paced digital era, the importance of data annotation tools cannot be overstated. With the explosion of visual content, companies are constantly seeking efficient ways to leverage this data for machine learning models. One of the standout innovations in this field is the *video labeling tool* that utilizes machine learning techniques. This article delves into the significance of such tools, the methodologies behind them, and how they are shaping the future of data annotation.
Understanding Video Labeling Tools
A video labeling tool is a software solution that helps annotate video data for machine learning purposes. It streamlines the process of marking different segments, objects, or actions within video content, providing invaluable training data for AI algorithms. The kernel of these tools lies in their ability to create structured datasets that enable deep learning models to learn from unstructured content.
Key Features of Video Labeling Tools
When choosing a video labeling tool, there are several features that can enhance the annotation process:
- Intuitive User Interface: Easy navigation and usability to ensure annotators can work efficiently.
- Support for Multiple Formats: Compatibility with various video formats and resolutions.
- Real-time Collaboration: Enables teams to work together in real-time and share annotations seamlessly.
- Automated Annotation: Leveraging machine learning algorithms to automate repetitive labeling tasks.
- Quality Control Tools: Features to validate annotations and ensure high-quality data output.
How Video Labeling Tools Enhance Machine Learning Models
The relationship between video labeling and machine learning is profound. Annotated video datasets serve as the foundation for training AI models that can recognize and interpret video content. Here are some ways video labeling tools boost the capabilities of machine learning frameworks:
1. Improved Accuracy in Object Detection
One of the primary applications of video labeling tools is in object detection tasks. By providing accurately labeled datasets, machine learning models can achieve a higher degree of accuracy. This is crucial for applications such as autonomous vehicle navigation, where recognizing objects like pedestrians and other vehicles in real-time can save lives.
2. Enhanced Action Recognition
Understanding the sequence of actions in a video can be complex. Video labeling tools help by allowing annotators to categorize actions within different timeframes. This categorization assists machine learning models in recognizing and predicting actions, largely benefiting industries like surveillance, sports analytics, and entertainment.
3. Efficient Training Processes
Developing robust machine learning models often involves training on vast amounts of data. Video labeling tools automate parts of the data preparation process, allowing for quicker dataset creation. As a result, data scientists can focus more on model development rather than data preparation.
Applications of Video Labeling Tools
The applications of video labeling tools extend across various industries. Here are some notable sectors where these tools are making an impact:
1. Autonomous Vehicles
The autonomous driving sector heavily relies on video labeling to train models that interpret real-world environments. By annotating thousands of videos, companies can teach vehicles to detect traffic signs, pedestrians, and obstacles effectively.
2. Sports Analytics
In sports, video labeling tools are used to analyze player movements, game strategies, and performance metrics. This data enables coaches to make informed decisions and improve player training regimens.
3. Healthcare
Healthcare applications involve using video labeling to analyze medical procedures or patient interactions. Annotated videos help in training models for diagnosing conditions or optimizing surgical outcomes through improved video analysis.
Why Choose Keylabs.ai for Video Annotation?
When it comes to finding the right partner for your video annotation needs, Keylabs.ai stands out as a premier data annotation platform. Here’s why:
1. Expertise in Machine Learning
With a dedicated team skilled in both machine learning and data annotation, Keylabs.ai can provide high-quality annotations that enhance your training dataset. This expertise ensures that your video labeling projects are executed with precision and care.
2. Scalable Solutions
Keylabs.ai offers scalable annotation solutions tailored to the size and complexity of your project. Whether you’re working with small datasets or large-scale video libraries, their platform can accommodate your needs effectively.
3. Advanced Annotation Tools
The video labeling tool at Keylabs.ai incorporates cutting-edge technology, such as automated annotations and real-time editing features, making the annotation process quick and efficient.
Best Practices for Using Video Labeling Tools
To maximize the benefits of video labeling tools, consider the following best practices:
1. Define Clear Annotation Guidelines
Establishing comprehensive annotation guidelines enhances the quality and consistency of annotated data. Clear directions help annotators understand key objectives and expectations.
2. Select the Right Tool for Your Needs
Each project may have different requirements. Select a video labeling tool that best fits the types of data you are working with and the outcomes you expect.
3. Regular Quality Control Checks
Conducting periodic reviews ensures that the annotations meet the expected standards. Incorporate feedback loops and revision stages to maintain high-quality output.
Future Trends in Video Labeling for Machine Learning
The landscape of video labeling is continuously evolving, thanks to advancements in machine learning and AI. Here are some anticipated trends:
1. Increased Automation
As machine learning algorithms become more sophisticated, we can expect a greater emphasis on automation in video labeling. Automated tools will help reduce human error and speed up the annotation process.
2. Enhanced Real-time Annotation Tools
Future tools will likely offer enhanced real-time annotation features, allowing teams to collaborate and annotate simultaneously, thus speeding up project completion times.
3. ASR and Voice Annotation
Voice recognition technology will be integrated into video labeling, enabling voice annotations that can provide context and additional information to annotated videos.
Conclusion
The video labeling tool is a game-changer in the realm of machine learning and data annotation. By providing structured, high-quality training datasets, these tools pave the way for innovative applications across various industries. Partnering with platforms like Keylabs.ai ensures that you harness the full potential of video data, driving progress in your AI initiatives. As the future unfolds, staying abreast of trends and embracing the innovations in video labeling will be crucial for any organization aiming to succeed in the competitive landscape of machine learning.
video labeling tool machine learning