Understanding Image Annotation for Machine Learning
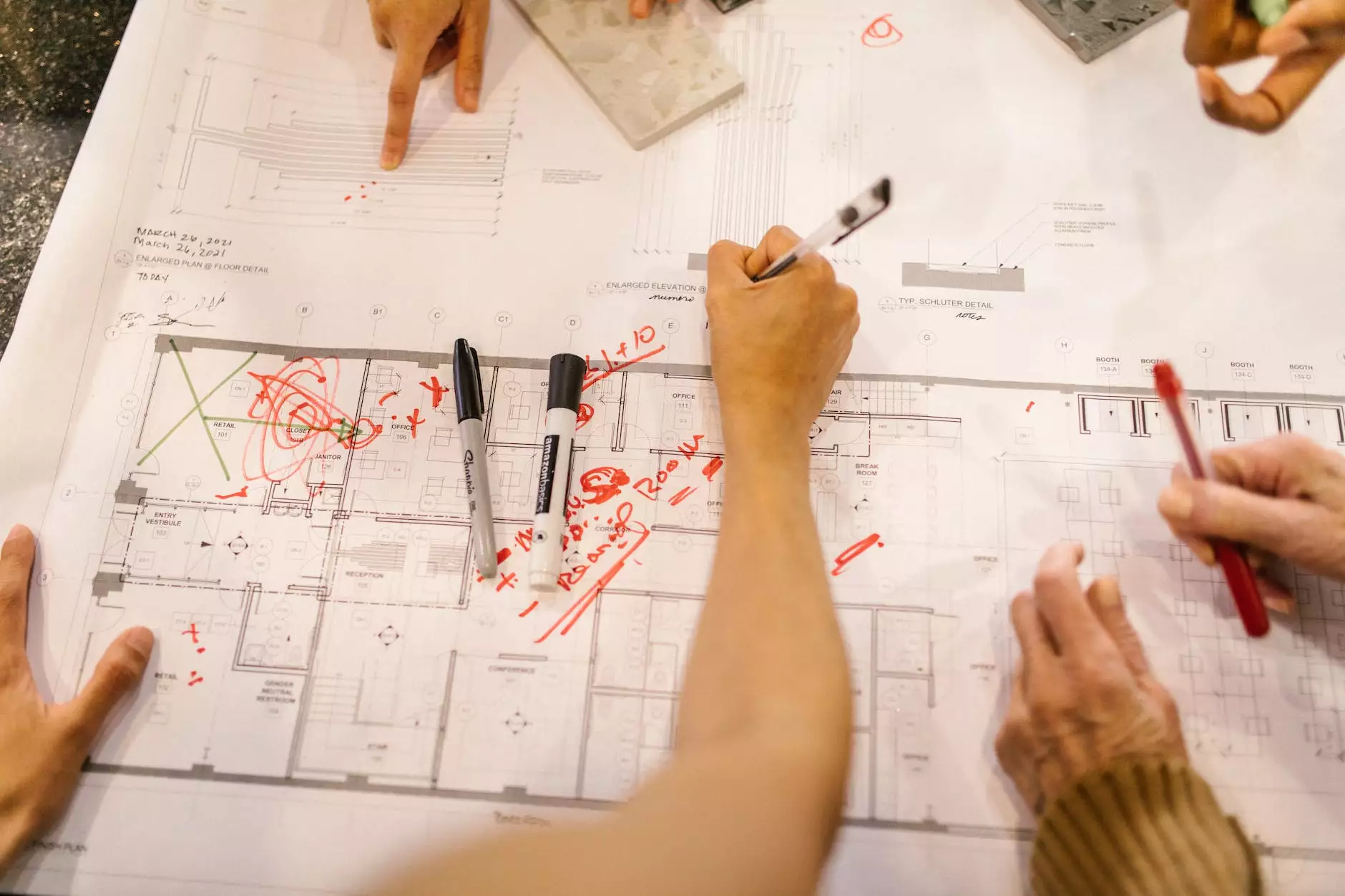
Image annotation for machine learning is an essential component that fuels the advancement of AI systems. It involves the process of labeling images to provide context and information necessary for training machine learning models. By enhancing the quality and quantity of labeled datasets, businesses position themselves to harness the true potential of AI technologies.
The Significance of Image Annotation
In the realm of artificial intelligence, particularly deep learning, the accuracy of data directly influences the performance of machine learning models. This is where the significance of image annotation for machine learning becomes apparent.
Why Businesses Require Image Annotation
- Model Accuracy: Annotated images lead to improved ability for models to learn from data, leading to more accurate predictions.
- Task-Specific Training: Various applications, from object detection to image segmentation, require tailored annotations to function optimally.
- Scalability: As businesses grow, the data they work with grows exponentially, necessitating efficient and scalable annotation solutions.
- Competitive Edge: High-quality datasets derived from proper image annotation confer a significant advantage in technological innovation.
The Image Annotation Process
The process of image annotation for machine learning can be broken down into several stages, each critical for producing high-quality labeled data.
Data Collection
The first step involves collecting relevant images that reflect the data needs of the project. These could include images from open datasets, company resources, or newly captured data that align with the intended application.
Choosing the Right Annotation Technique
There are various methods of annotation that can be utilized, including:
- Bounding Boxes: Ideal for object detection tasks. Rectangular boxes are drawn around the object of interest.
- Semantic Segmentation: Involves labeling each pixel of an image, making it suitable for tasks requiring detailed context.
- Instance Segmentation: Combines object detection and semantic segmentation, distinguishing between different instances of the same object.
- Landmark Annotation: Used for facial recognition or action recognition, marking specific points of interest on an image.
Implementing an Effective Annotation Strategy
To maximize the benefits of image annotation for machine learning, businesses should implement a strategic approach:
1. Define Objectives Clearly
Understanding the end goal of the machine learning model is crucial. Defining clear objectives will guide the annotation style and the complexity required.
2. Leverage Technology
Using advanced annotation tools can significantly streamline the process:
- Automated Annotation Tools: Software that uses AI to assist in the annotation process can significantly reduce time and labor costs.
- Quality Assurance Mechanisms: Implementing a review system ensures that the annotations meet high standards.
- Collaboration Features: Tools that allow multiple users to annotate simultaneously can increase efficiency.
3. Engage Skilled Annotators
Whether using an in-house team or outsourcing to a specialized service like Keylabs.ai, having skilled annotators is vital. They must understand the nuances of the data domain and be trained in the chosen annotation techniques.
Challenges in Image Annotation
Despite its importance, image annotation for machine learning does come with challenges, including:
1. Scalability
As datasets grow, managing the increased demand for annotation can become cumbersome. Businesses need scalable solutions that can grow alongside their data needs.
2. Cost Efficiency
High-quality annotation can be resource-intensive. Balancing quality with cost-efficiency is essential to maintain the project ROI.
3. Consistency
Maintaining consistency across annotations is critical. Any discrepancies can lead to biased models. Therefore, having clear guidelines and review processes is crucial.
Benefits of Using Keylabs.ai for Image Annotation
Choosing the right partner for data annotation is vital. Keylabs.ai offers a robust platform that excels in providing comprehensive data annotation solutions. Here’s why it stands out:
1. Advanced Technology
Keylabs.ai utilizes cutting-edge technologies that enhance the annotation process, ensuring high precision and efficiency.
2. Expertise
With a team of seasoned professionals and subject matter experts, Keylabs.ai guarantees that every annotation is performed to the highest standards.
3. Customizable Solutions
The platform offers tailored annotation services that adapt to your specific project needs, allowing for flexibility and relevance.
4. Quick Turnaround Times
Speed is crucial in the fast-paced world of machine learning. Keylabs.ai ensures that annotated data is delivered promptly without compromising quality.
Future of Image Annotation in Machine Learning
The landscape of image annotation for machine learning is continually evolving, with new technologies and methodologies emerging. Here are some trends to watch for:
1. Increased Automation
With the rise of AI technologies, we can expect a greater level of automation in the annotation process, making it faster and more efficient.
2. Enhanced Collaboration
As remote work becomes more common, collaborative platforms that allow for real-time annotation and feedback will grow in demand.
3. Continuous Learning Systems
Annotation tools that leverage machine learning to improve over time will enhance productivity, allowing models to learn from past errors.
Conclusion
In conclusion, image annotation for machine learning forms the backbone of developing robust AI models. By investing in quality annotation processes through advanced platforms like Keylabs.ai, businesses can harness the full potential of their data, driving innovation and maintaining a competitive edge in an ever-evolving landscape.
To stay ahead of the curve, organizations must prioritize their data strategies, integrating effective annotation techniques that align with their broader goals. The future of AI is bright, and proper image annotation is the key to unlocking its full potential.